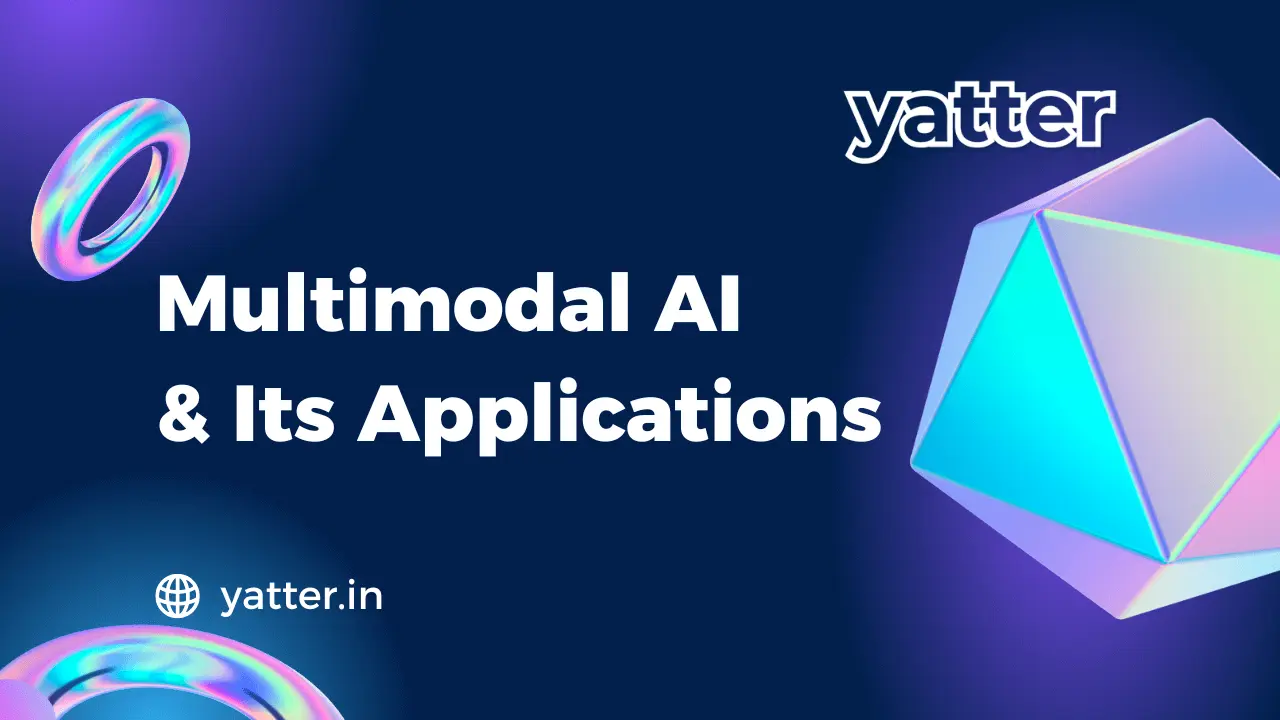
Overview
Multimodal AI is a helper that can understand different things all at once. It can understand words, pictures, and even how we talk! Imagine showing it a picture, and it can tell you what’s in it. Or speaking to it, and it knows exactly what you’re asking for! This kind of AI makes our gadgets really smart and helps us talk to them in a way they understand. It’s like having a friendly assistant who always knows what we need.
In this blog, we will search deeper into the complexity of Multimodal AI, exploring its underlying principles, diverse applications, and the impact it has on shaping the future of technology. Join us on this journey as we unravel the potential of Multimodal AI to revolutionize the way we interact with and harness the power of information in the digital age.
Understanding Multimodal AI
Unimodal AI focuses on analyzing data within a single mode, like text, images, audio, or video. For instance, a text-based AI system might analyze written content, while an image-based one could classify images.
Multimodal AI goes beyond this by integrating multiple modes of data. Instead of working separately, it combines different streams of data to gain deeper insights and provide more context. By using text, images, sound, and sensor data together, Multimodal AI can understand the world more comprehensively, similar to how humans do.
Examples of multimodal AI:
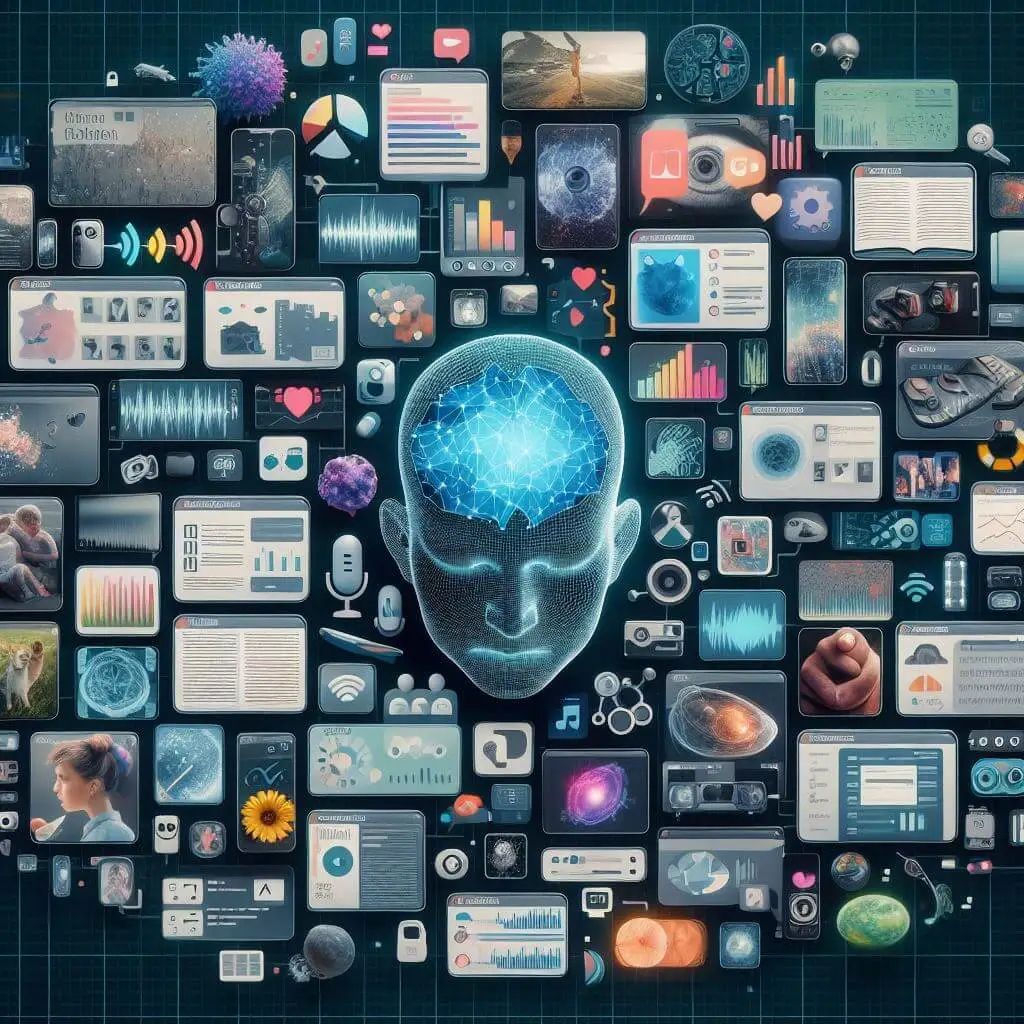
Multimodal data encompasses a variety of formats, each offering unique insights and perspectives. Textual data, such as articles, emails, and social media posts, conveys information, emotions, and opinions. Images, ranging from photographs to medical scans, provide rich visual context for analysis. Audio data, including speech, music, and ambient noise, adds another layer of context by conveying spoken language and emotions. Video data combines images with accompanying audio to create dynamic visual experiences, including movies, surveillance footage, and live streams, offering a comprehensive view of events, interactions, and environments. By integrating these diverse modalities of data, Multimodal AI can unlock new capabilities and insights that transcend the limitations of individual data types, revolutionizing various fields and enhancing human-machine interaction.
Applications of Multimodal AI
- Image Captioning:
Image captioning is a compelling application of Multimodal AI that involves generating descriptive captions for images. By combining image analysis with natural language processing (NLP) techniques, Multimodal AI systems can understand the visual content of an image and generate coherent textual descriptions. This capability finds applications in areas such as content accessibility, image indexing, and enhancing user experiences in image-based platforms. - Speech Recognition:
Multimodal AI plays a crucial role in advancing speech recognition technology by integrating audio data with contextual information from other modalities. By analyzing both the acoustic features of speech and the accompanying textual or visual context, Multimodal AI systems can improve the accuracy and robustness of speech recognition algorithms. This enables applications such as voice-controlled assistants, dictation software, and voice search functionalities. - Video Understanding:
Video understanding involves extracting meaningful insights from video data by combining visual and auditory cues with contextual information. Multimodal AI enables machines to analyze video content, identify objects, actions, and events, and understand the temporal relationships between different elements. Applications of video understanding powered by Multimodal AI include video summarization, content recommendation, surveillance analysis, and activity recognition. - Multimodal Translation:
Multimodal AI facilitates more accurate and contextually relevant translation by integrating multiple modalities of data, such as text, images, and audio. By considering visual and auditory cues alongside textual input, Multimodal AI systems can enhance translation quality and address challenges like ambiguity and cultural nuances. This enables applications such as multilingual image translation, sign language interpretation, and cross-modal document translation. - Virtual Assistants:
Virtual assistants leverage Multimodal AI capabilities to understand and respond to user queries across various modalities, including text, speech, and images. By integrating natural language understanding, speech recognition, and computer vision technologies, virtual assistants can provide personalized and contextually relevant assistance in tasks ranging from scheduling appointments to retrieving information from diverse sources. Multimodal AI-powered virtual assistants enhance user productivity and streamline interactions in both personal and business settings. - Yatter AI: Integration of Multimodal Capabilities for Enhanced User Experience:
Yatter AI represents a paradigm shift in intelligent virtual assistance by seamlessly integrating Multimodal AI capabilities into user interactions. By processing text, images, audio, and video data, Yatter AI offers versatile assistance, from answering questions to solving complex problems. Its ability to understand and respond to multimodal inputs enables more natural and contextually relevant interactions, enhancing user satisfaction and productivity. Whether retrieving information from images, transcribing voice messages, or providing personalized recommendations, Yatter AI leverages Multimodal AI to deliver a seamless and intuitive user experience.
Challenges and Limitations of Multimodal AI
- Data Integration:
Data integration is a key challenge in Multimodal AI, involving the harmonization of diverse sources of multimodal data for analysis. This process requires preprocessing, alignment, and synchronization to ensure consistency and coherence. Multimodal datasets vary in format, quality, and scale, adding complexity to integration efforts. Robust pipelines, alignment algorithms, and scalable infrastructure are needed to handle these challenges effectively. - Model Complexity:
Multimodal AI models are inherently more complex than unimodal ones due to the need to process and integrate multiple modalities of data. Designing and training such models requires careful consideration of architecture, parameterization, and optimization techniques for optimal performance. As the number of modalities and interactions between them increases, so do computational and memory requirements. Balancing complexity with efficiency and scalability is a major challenge in Multimodal AI research. - Computational Resources:
Multimodal AI tasks demand significant computational resources to process data from multiple modalities simultaneously. This includes CPU and GPU resources for training and inference, as well as storage and memory resources for large datasets and model parameters. Scaling up resources to meet these demands can be costly and challenging, particularly for organizations with limited infrastructure or budget constraints. Optimizing resource utilization and minimizing latency further complicate computational resource management.
How Multimodal AI Works
Multimodal AI is an advanced technology that combines different modes of input, such as text, images, and audio, to understand and generate responses. Here’s a simplified explanation of how it works:
1. Input Gathering:
The process begins with collecting various types of input, which could include text, images, audio, or a combination of these. This step is crucial as it provides the necessary data for the AI system to analyze and understand.
2. Safety Mechanisms:
Before processing the input, safety mechanisms are often implemented to ensure that the content is appropriate and meets certain standards. These mechanisms may filter out offensive or sensitive material to maintain a safe and respectful environment.
3. Model Processing:
Once the input is gathered and filtered, it undergoes processing by the AI model. This involves utilizing sophisticated algorithms and deep learning techniques to analyze the data and extract relevant information. The AI model is trained on vast amounts of data to recognize patterns and make accurate predictions.
4. Output Processing:
After processing the input, the AI system generates an output based on the analyzed data. This output could take various forms depending on the nature of the input and the task at hand. For example, it could be a text response, an image classification, or a synthesized voice message.
5. Output Delivery:
The final step involves delivering the output to the user or system that initiated the request. This could be through a user interface, such as a chatbot or a mobile app, or through an API for integration with other software systems. The goal is to provide the user with relevant and meaningful information in a timely manner.
Multimodal AI works by collecting input from different sources, processing it using advanced algorithms, and generating an appropriate output based on the analyzed data. It enables AI systems to understand and respond to human input in a more natural and versatile way, opening up new possibilities for interaction and problem-solving.
Conclusion
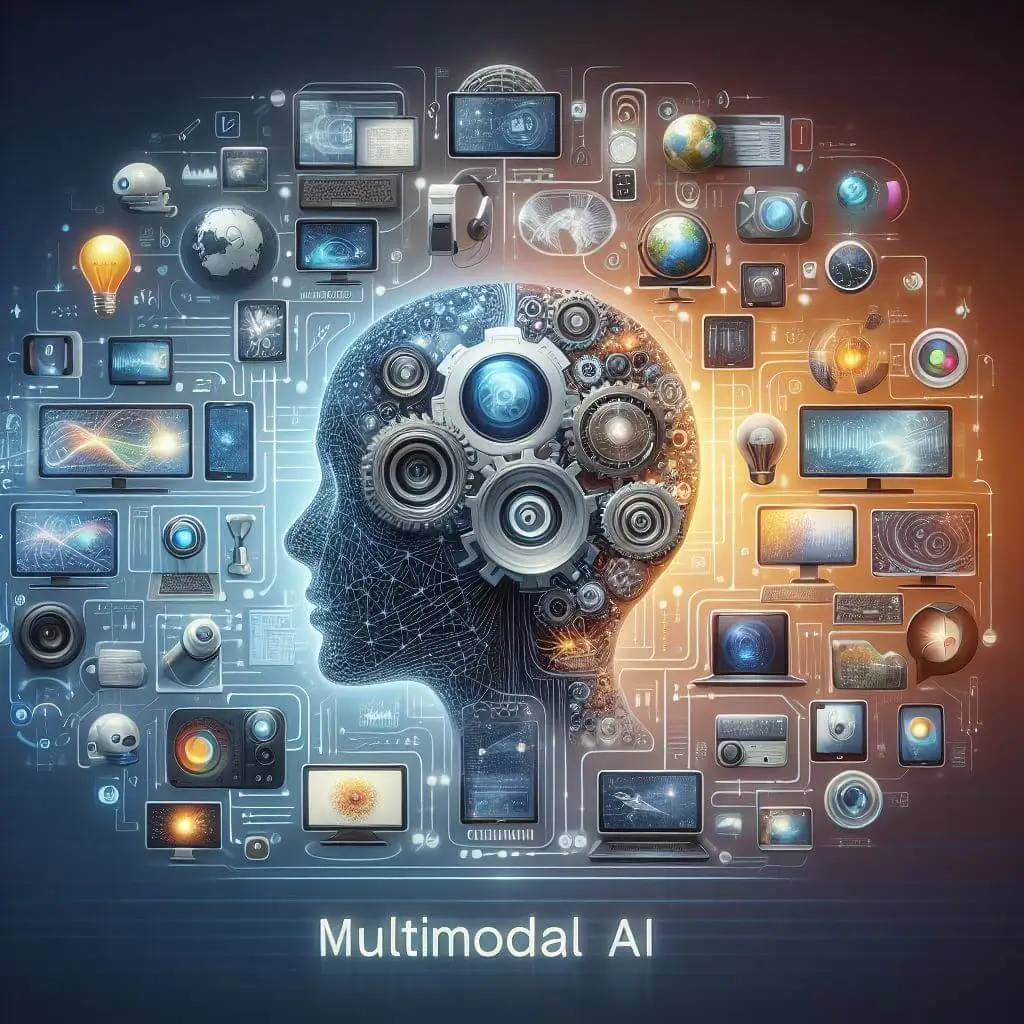
In summary, Multimodal AI represents a significant leap forward in artificial intelligence, enabling machines to process and understand information from various modalities like text, images, audio, and video. This integration of diverse data sources enhances our ability to perceive and interact with the world more comprehensively. Multimodal AI finds applications in image captioning, speech recognition, video understanding, and virtual assistants, revolutionizing communication, collaboration, and innovation in the digital age.
Looking ahead, there’s vast potential for advancing Multimodal AI through research and development. Progress in deep learning architectures, transfer learning, and cross-modal retrieval is paving the way for more robust systems. It’s crucial to deploy these technologies responsibly, considering ethical implications and societal concerns.
Yatter AI emerges as a promising platform for leveraging Multimodal AI, especially in intelligent customer support and information retrieval. Its ability to integrate multimodal capabilities offers personalized assistance across various channels. As Multimodal AI evolves, Yatter AI’s potential to enhance customer experiences and drive innovation remains unmatched.
In conclusion, the journey of Multimodal AI is marked by innovation and collaboration. By embracing responsible development practices, we can unlock new opportunities for intelligent interaction, shaping a future where humans and machines work together seamlessly to tackle tomorrow’s challenges.